Technology in Retail : The Story So Far
Retail is a legacy industry and just like most legacy industries, technology is the greatest enabler in modern times. Technology-led optimisation of the retail value chain is not just limited to online businesses but also applies to core retail functions like inventory management, warehousing, buying and merchandising.
Post the COVID-19 pandemic, consumer baskets like Europe discovered the vulnerabilities of physical retail. Omnichannel models were largely preferred and a lot of large-scale retailers revamped their tech stacks to accommodate the new world.
In-store buying returned to peak levels after the end of the pandemic. The technology adopted during the pandemic only helped boost profitability. There were large gaps in the supply chain across the European markets and optimizing for the same led to better margins and growth even as the consumer’s spending dwindled for a while.
The bottomline gains compensated for the topline losses that were triggered by tumultuous times for retailers that were quick to adapt.
How Generative AI impacted retail?
The metaverse was a passing trend. Digital storefronts were cosmetic changes.
However, some applications such as personalisation of a user’s shopping experience.
Interactive chatbots, virtual trial rooms found relevance with the gen Z consumer.
The explosion of Open AI’s chat GPT, just made it easier for technology based businesses to include these technologies into their retail stacks. A number of softwares mushroomed. Internal technology teams were also quick to adopt to these powerful LLMs and build applications on the top.
All they needed was a strong data foundation. The quality of their first party data determined the performance of the adopted technologies or the homegrown applications built on top.
As a retail technology company born out of the largest retailer in India we did a study of the most popular applications built on LLMs and generative AI. And here’s a list.
We also compared AGI applications to EGI ( Enterprise General Intelligence) applications to understand the benefits and disadvantages of the system. Read on to know more :
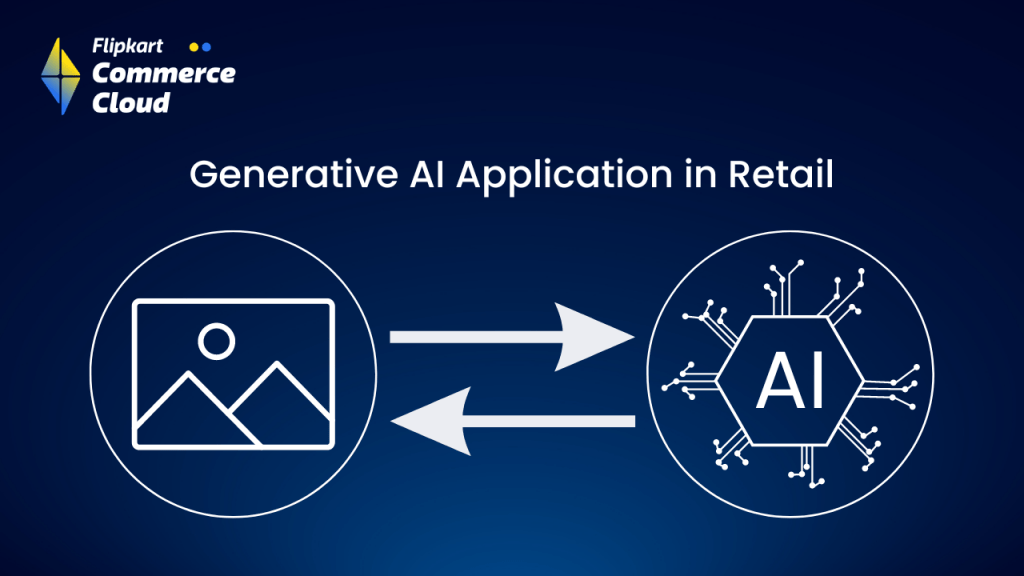
The most popular Generative AI applications in Retail
- Personalized Product Recommendations:
Generative AI can analyze customer behavior, preferences, and past purchases to generate highly personalized product recommendations. This technology takes into account various data points and uses advanced algorithms to suggest products that a customer is likely to be interested in. This enhances the customer shopping experience and can increase conversion rates for retailers. - Virtual Try-On Solutions:
Virtual try-on solutions powered by generative AI allow customers to visualize how clothing, accessories, or even makeup products would look on them before making a purchase. By analyzing customer images or videos, these technologies can simulate the appearance of the product on the individual, helping customers make more informed decisions and reducing the rate of returns. - Custom Product Design:
Some retailers use generative AI to allow customers to customize products to their preferences. For example, customers can design their own sneakers, furniture, or jewelry by interacting with a generative design tool that takes their inputs and generates unique product designs. - Inventory Management and Demand Forecasting:
Generative AI can help retailers optimize their inventory management by analyzing historical sales data, market trends, and external factors to forecast future demand more accurately. This can lead to reduced overstocking or understocking issues, resulting in improved supply chain efficiency.
How is the Retail industry using LLMs?
- Customer Support and Chatbots:
LLMs were being employed to develop advanced chatbots for customer support. These chatbots could understand customer inquiries and provide accurate and helpful responses, enhancing the customer experience by providing instant assistance 24/7. - Product Descriptions:
LLMs were employed to create detailed and compelling product descriptions. These descriptions could highlight features, benefits, and use cases for products, helping customers make informed purchasing decisions. - Market Research and Trend Analysis:
LLMs were used to analyze social media trends, customer reviews, and online discussions to gather insights about consumer preferences and emerging trends. This information could guide retailers in product development and marketing strategies. - Virtual Shopping Assistants:
Some retailers were experimenting with LLM-powered virtual shopping assistants that could interact with customers in a more natural and conversational manner. These assistants could help customers find products, compare options, and answer questions. - Localized Marketing and SEO:
LLMs were used to generate localized content and improve search engine optimization (SEO) efforts. Retailers could create content that resonated with specific regions and local audiences, improving their online visibility - Enhanced Product Search: LLMs were employed to enhance on-site search functionality. By understanding natural language queries, these models could improve the accuracy of search results and help customers find products more efficiently.
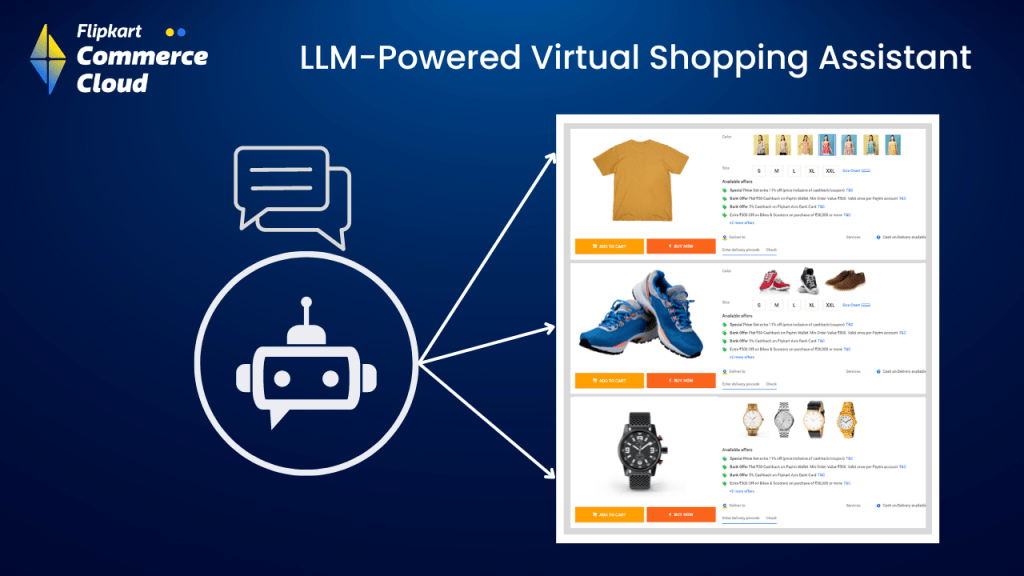
But what about domain specific models ? Are they Passe?
No, this is the simple answer. Domain specific intelligence or Enterprise general intelligence will still be required in very intricate functions.
The more variance in a general model the better, but certain critical functions require more bias in ensuring smooth operations and critical checks. Domain specific intelligence is still necessary and will be required in building technology for finance, legal , and microeconomics.
You just can’t make do with a college graduate or a post graduate in business, in some domains, you need expert counsel and consultation. Similarly some critical functions will require a closed training data set and very specific answers.
Also the ‘ AI hallucination’ does not inspire a lot of confidence in using Open source LLMs for the more critical functions. All these general query and NLP applications are still built atop very specific datasets to give the best results.
Bonus Read :
The Data Problem | How did Alpaca match the results generated by Chat GPT?
Models designed to follow instructions, like GPT-3.5 (text-davinci-003), ChatGPT, Claude, and Bing Chat, have gained substantial capabilities. These models are now commonly interacted with by users, often even in professional settings. Despite their widespread use, these instruction-following models still possess certain shortcomings: they can generate inaccurate information, perpetuate societal biases, and produce offensive language.
To effectively tackle these critical issues, it is imperative for the academic community to participate. Regrettably, conducting research on instruction-following models in an academic context has been challenging due to the absence of an easily accessible model that matches the capabilities of proprietary models like OpenAI’s text-davinci-003.
Stanford labs therefore developed an instruction-following language model named Alpaca. This model is fine-tuned from Meta’s LLaMA 7B model. Alpaca is trained on 52K instances of instruction-following demonstrations, created in a manner similar to self-instruction using text-davinci-003. When evaluated against the self-instruct benchmark, Alpaca exhibits behaviors reminiscent of OpenAI’s text-davinci-003, while also being surprisingly compact and straightforward to reproduce at a low cost.