The landscape of pricing has evolved far beyond the days of straightforward cost-plus calculations or mimicking competitors’ pricing. In this era of big data and advanced technology, retailers have a powerful tool at their disposal – Machine Learning. In this blog post, we’ll take a close look at how machine learning can change the way retailers set their prices.
Limitations of Traditional Price Optimization Methods
Before we delve into machine learning-based price optimization, it’s crucial to understand the limitations of traditional methods. Traditionally, retailers often relied on rule-based approaches without advanced AI algorithms or simple cost-plus pricing models to set their product prices. While these methods have their merits, they come with significant drawbacks:
1. Lack of Precision
Traditional pricing methods are typically based on historical data, cost structures, and market benchmarks. They lack the precision needed to account for the dynamic and multifaceted nature of today’s markets. As a result, prices may not accurately reflect consumer demand or competitive dynamics.
2. Inability to Adapt Quickly
Market conditions change rapidly, especially in the digital age. Traditional pricing models are often too slow to adapt to these changes. Retailers may miss out on opportunities for profit maximization or risk pricing themselves out of the market.
3. Neglecting Customer-Centric Pricing
Modern consumers are more demanding and price-conscious than ever. Traditional pricing models tend to overlook the individual preferences and willingness to pay of customers. Machine learning, on the other hand, excels at personalizing prices to individual customer segments.
This is where modern technologies like AI and Machine Learning come into play.
What Is Machine Learning Based Price Optimization?
Machine learning-based price optimization is a cutting-edge approach that revolutionizes how retailers determine the optimal prices for their products. At its core, it’s a data-driven methodology that harnesses the power of advanced algorithms and artificial intelligence to analyze vast datasets encompassing historical sales, customer behavior, competitor pricing, and external market factors. Unlike traditional static pricing methods, machine learning dynamically adjusts prices in real-time, responding to changing market conditions and customer preferences.
This approach not only ensures that prices remain competitive but also allows for personalized pricing, catering to the unique needs of individual customers or segments. Furthermore, ML continuously refines pricing strategies through automation and data-driven insights, reducing human bias and inefficiencies.
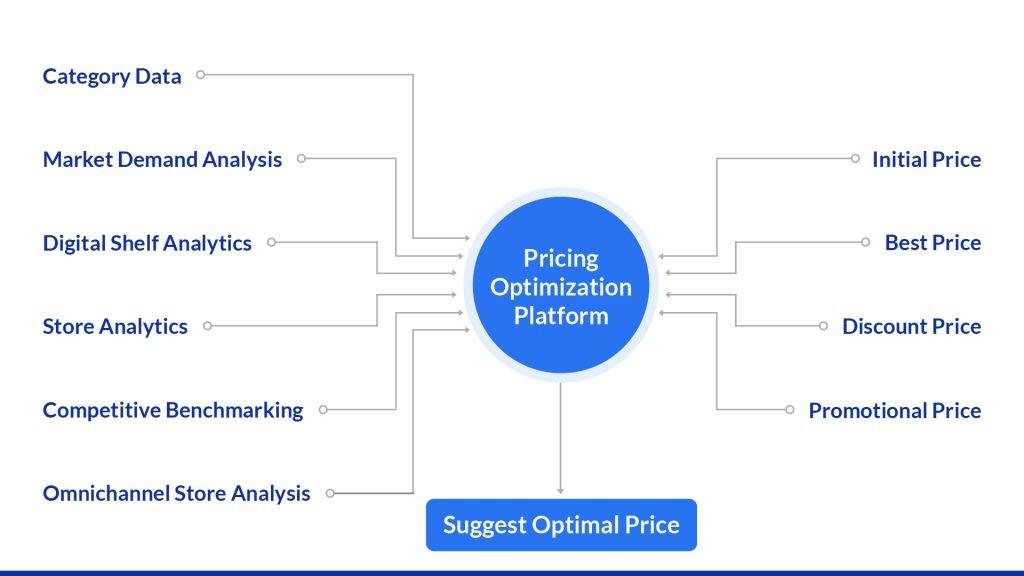
How Machine Learning Can Improve Pricing Performance
Machine learning-based pricing overcomes the limitations of traditional methods by harnessing the power of data and advanced algorithms. Here are some ways in which it can help retailers set the right price:
Data-Driven Decision Making: Machine learning-based pricing relies on a wealth of available data, including historical sales, market trends, and customer behavior. By analyzing this available data, machine learning algorithms can identify patterns and relationships that are virtually impossible for humans to discern. This data-driven approach ensures that pricing decisions are based on concrete evidence rather than guesswork.
Dynamic Pricing: One of the key advantages of machine learning is its ability to implement dynamic pricing. This means that prices can be adjusted in real-time based on a variety of factors, such as demand fluctuations, competitor pricing changes, and even weather conditions. This agility ensures that prices remain competitive and maximize revenue potential.
Personalization: Machine learning doesn’t treat all customers the same. It can segment customers based on their preferences, buying history, and price sensitivity. This segmentation enables retailers to offer personalized prices to different customer groups, enhancing customer satisfaction and loyalty.
Optimization: Machine learning algorithms are designed to optimize pricing strategies continuously. They analyze data and adjust prices to achieve specific objectives, whether it’s maximizing revenue, profit margins, or market share. This ongoing optimization ensures that retailers are always on the path to achieving their financial goals.
Reduced Human Bias: Human bias can often creep into pricing decisions, whether it’s due to gut feelings, heuristics, or personal preferences. Machine learning mitigates this bias by making decisions purely based on data, leading to more objective and rational pricing choices.
Accurate Predictions: Perhaps the most compelling aspect of machine learning-based pricing is its ability to accurately predict the optimal price. By considering numerous variables and constantly refining its models, machine learning can pinpoint the price point that will yield the best results, whether that means maximizing revenue or profit.
While machine learning-based pricing is a powerful tool for retailers, it should be seen as a complement to human expertise rather than a replacement. Humans bring invaluable qualities like judgment, adaptability, ethical considerations, and the ability to handle unforeseen circumstances. A successful pricing strategy should strike a balance between data-driven insights and human intervention to achieve the best results while maintaining trust and ethical standards.
How Machine Learning Models Deal with Data
Machine learning models employ various strategies to deal with data intricacies and uncertainties:
Working with Existing Data
When it comes to working with existing data, ML models go beyond mere data storage and retrieval. They employ advanced techniques such as feature engineering and dimensionality reduction to extract meaningful insights from the data. Feature engineering involves selecting and transforming relevant variables to create new features that improve model accuracy. Dimensionality reduction techniques like Principal Component Analysis (PCA) help simplify complex datasets, making them more manageable for analysis.
Machine learning models excel at identifying non-linear relationships within the data. This means they can capture intricate patterns that traditional linear models might miss. For example, they can discern the impact of interactions between variables on pricing decisions, uncovering hidden insights that can drive more effective pricing strategies.
Loss Data Simulation
One of the most compelling aspects of machine learning-based price optimization is its capacity for loss data simulation. Traditional methods seldom offer a way to experiment with different pricing strategies without risking actual revenue. In contrast, machine learning models can simulate countless pricing scenarios, allowing retailers to explore various strategies in a risk-free environment.
These simulations not only provide insights into potential gains but also shed light on worst-case scenarios. Retailers can assess the impact of aggressive price cuts, competitor reactions, and even external events like economic downturns. Armed with this knowledge, they can make informed decisions to mitigate risks and maximize rewards.
The Predictive Pricing Model
At the core of ML-based price optimization lies the predictive pricing model. What sets this model apart is its adaptability and ability to incorporate real-time data. As new sales data, competitor pricing information, and other relevant factors become available, the model continuously updates its predictions.
This dynamic nature enables online retailers to respond swiftly to changing market conditions for different goods. For instance, if a sudden surge in demand occurs due to an unexpected event, the model can adjust prices in real-time to capitalize on the opportunity. Conversely, if demand wanes, the model can implement targeted discounts to stimulate sales of different goods without sacrificing profitability.
Furthermore, machine learning models can handle multivariate forecasting, considering multiple factors simultaneously. This is crucial in today’s retail environment, where customer behavior is influenced by a myriad of factors, from promotions to seasonality to external events. These models excel at deciphering complex relationships and providing nuanced insights into how various factors interplay to affect pricing outcomes.
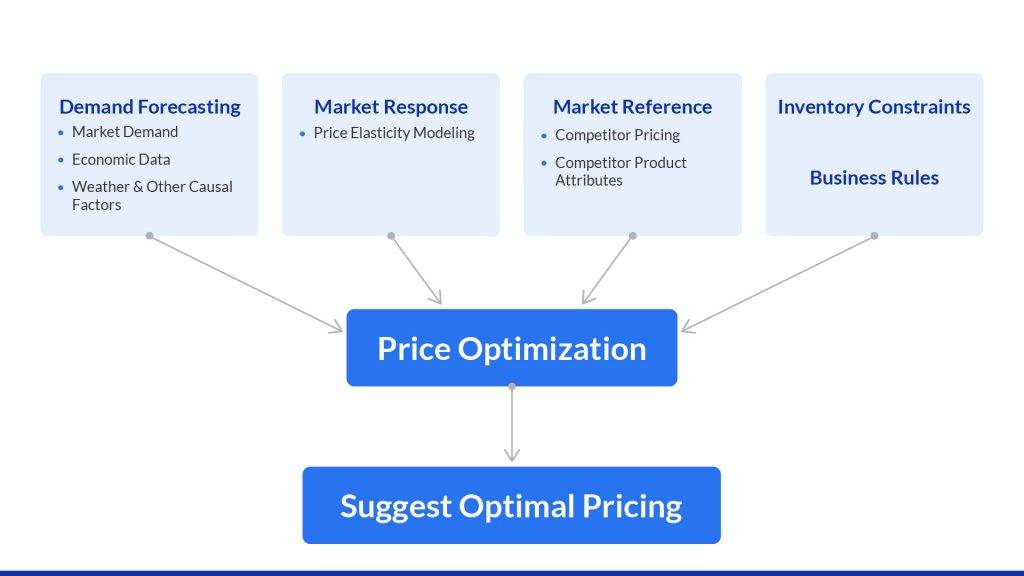
The KNN Method (K-Nearest Neighbors)
The KNN method, a ML technique, adds a collaborative dimension to price optimization. It goes beyond simple data analysis by identifying similarities between products or scenarios. By considering the pricing history of similar products, KNN leverages collective experiences to guide pricing decisions.
What’s fascinating about KNN is its ability to learn from the collective wisdom of the entire product catalog. It’s akin to tapping into the expertise of every product category, rather than relying on isolated data points. For example, if a retailer is introducing a new product, KNN can analyze the pricing strategies that have been successful for similar products in the past, providing valuable guidance for setting an optimal price.
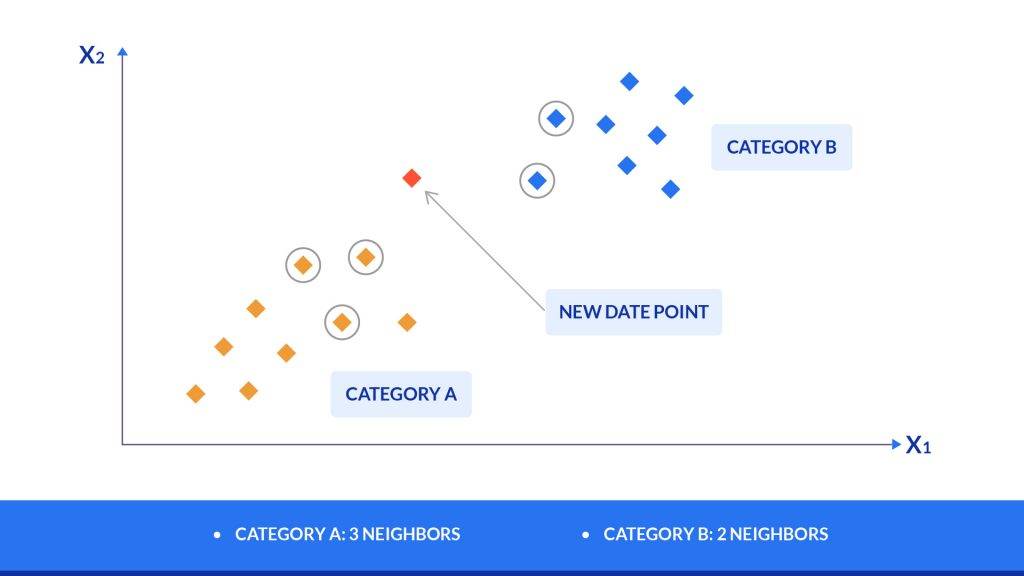
Explore Exploit Algorithm to React with Agility
While setting up dynamic pricing, analyzing historical data is not enough. Businesses need robust algorithms that can adapt swiftly to the dynamic changes in the market. This is where the Explore-Exploit machine learning algorithm comes into play. These algorithms keep a balance between exploring new possibilities while exploiting the already known information based on historical data.
By leveraging the Explore-Exploit algorithm, businesses can test various pricing approaches to identify optimal pricing, optimize existing strategies, and quickly adjust pricing to capitalize on emerging trends. Moreover, with time, these algorithms learn from the outcome and use the knowledge to help retailers make precise pricing decisions.
Benefits of Machine Learning-Based Pricing Models
Among the myriad advantages offered by ML-based pricing models, several standout prominently. Let’s delve into some of these notable benefits.
Deep Analysis of Huge Amounts of Data
Machine learning pricing models excel at processing and analyzing vast datasets. Traditional pricing models often rely on historical data, limited in scope and timeliness. Machine learning, on the other hand, can ingest and analyze real-time data from various sources like sales transactions, competitor pricing, and market trends.
This deep analysis allows businesses to uncover hidden patterns and correlations that were previously overlooked. By considering numerous variables simultaneously, such as demand, seasonality, and consumer behavior, machine learning models can make more accurate price predictions, leading to better revenue optimization.
Better Assortment Management
Machine learning pricing models extend their influence beyond just pricing. They also assist in assortment management. By examining purchasing patterns and consumer preferences, these models help businesses identify which products should be bundled together or promoted as cross-sells. This leads to a more effective product mix, improved inventory management, and ultimately higher profitability.
Furthermore, these models can optimize inventory levels, reducing the risk of overstocking or understocking. This level of precision is unattainable with traditional pricing approaches.
Avoid Profit Loss Due to Excessive Discounting
Excessive discounting can be a significant source of profit loss for businesses. Machine learning pricing models are equipped with the capability to identify the optimal discount level for each product in real-time. By considering factors such as historical sales data, competitor pricing, and current market conditions, these models can suggest discounts that maximize revenue while preserving profit margins.
This prevents businesses from falling into the trap of over-discounting, which erodes profits without necessarily driving additional sales. It ensures that discounts are applied strategically to achieve specific business goals.
Overcome Traditional Pricing Limitations
Traditional pricing methods, often based on cost-plus or competitor-based strategies, have limitations that hinder their effectiveness. Machine learning pricing models are not bound by these limitations. They can take into account a myriad of dynamic factors, such as customer segmentation, pricing elasticity, and even weather conditions, to make real-time pricing decisions.
This adaptability allows businesses to respond rapidly to changing market conditions and consumer behavior, staying ahead of the competition and maximizing profitability.
Better Understanding of Consumer Behavior and External Factors
One of the most significant advantages of machine learning-based pricing models is their ability to gain insights into consumer behavior and external factors that impact pricing decisions. By analyzing data from various touchpoints, such as online interactions, social media sentiment, and economic indicators, these models can identify trends and correlations that influence purchasing behavior.
This deeper understanding enables businesses to tailor their pricing strategies to specific customer segments, personalize promotions, and predict consumer responses to different pricing scenarios. It also helps them proactively adjust prices in response to external factors like economic downturns, supply chain disruptions, or changes in competitor pricing.
Examples of Businesses Harnessing Machine Learning for Price Optimization
The application of machine learning in price optimization has not only gained traction but has also delivered significant successes across various industries. Let’s delve into some compelling examples that highlight how businesses have harnessed the power of machine learning to transform their pricing strategies.
Hospitality Industry: Hilton and InterContinental Hotels Group
In the early 2000s, Hilton Hotels Corp and InterContinental Hotels Group embarked on a pioneering journey by replacing fixed room rates with a dynamic pricing scheme. Back then, room rates were adjusted once or twice a day. Today, thanks to advancements in computational power, prices can change almost in real-time.
Dynamic pricing remains a cornerstone of the hotel industry, heavily reliant on machine learning. Airbnb, for instance, offers hosts a dynamic pricing tool that suggests rates based on parameters like seasonality, day of the week, special events, and even sophisticated factors like property photos and neighborhood pricing trends. The success of this approach has inspired other major players, such as eBay and Uber, to adopt similar strategies.
eBay: Dynamic Pricing and Auction Optimization
eBay, a global online marketplace, uses machine learning to optimize pricing for both fixed-price listings and auctions. Their algorithms take into account various factors like historical sales data, competitor prices, and user behavior to recommend starting prices for auctions and suggest price adjustments for fixed-price listings. This ensures that sellers on eBay have competitive pricing while maximizing their chances of selling items at the best possible price.
Brick-and-Mortar Retail: Ralph Lauren, Michael Kors, and Zara
While e-commerce has seen substantial advancements in machine learning-driven price optimization, brick-and-mortar retailers are also making strides. Companies like Ralph Lauren and Michael Kors have employed machine learning to reduce the need for frequent markdowns and enhance inventory management. Their aim is to boost profit margins, even if it means sacrificing some revenue.
Zara, a prominent fashion retailer, relies on machine learning to minimize promotional activity and swiftly adapt to evolving fashion trends. These examples illustrate that traditional retail can also reap the rewards of machine learning-driven pricing strategies.
The Future of Pricing is Driven by Machine Learning
The future of pricing, undoubtedly, is being reshaped by machine learning. This groundbreaking technology has the potential to not only optimize pricing but also unlock new levels of precision, responsiveness, and profitability for businesses across various industries.
And one standout player in this field is Flipkart Commerce Cloud.
Powered by advanced machine learning algorithms, FCC’s price optimization engine is designed to unlock the full potential of your pricing strategy. Our proprietary model takes into account intricate details, such as demand elasticity and game theory principles, to deliver pricing decisions that drive profitability and customer satisfaction. With a proven track record across millions of SKUs and diverse product categories, our engine ensures precision in pricing adjustments, achieving impressive business impacts.
By leveraging the power of data and seasoned experts, we provide our clients with a competitive edge, making FCC’s price optimization engine an indispensable tool for modern businesses looking to thrive in the dynamic world of pricing strategies.
Ready to revolutionize your pricing strategy with FCC’s machine learning expertise? Talk to our experts today.
FAQs
What kind of data is needed for machine learning price optimization?
For machine learning price optimization, you need historical data on prices, sales, customer behavior, market trends, and any other relevant factors that may impact pricing. This data is used to train the machine learning algorithms to make accurate predictions and optimize prices for maximum profitability.
What are the limitations of using machine learning for price optimization?
Some limitations of using machine learning for price optimization include the need for large amounts of high-quality data, potential bias in the data that can lead to inaccurate predictions, and the challenges in interpreting and explaining the decisions made by machine learning models to stakeholders.
Can machine learning be combined with other pricing strategies, such as dynamic pricing or value-based pricing?
Yes, machine learning can be combined with other pricing strategies like dynamic pricing or value-based pricing. By leveraging machine learning algorithms and real-time data, businesses can optimize prices based on market demand, customer behavior, and other factors to maximize revenue and profitability.
Recommended Read:
1. Price optimization model: How retailers can use it for business growth