In an era where market landscapes evolve at the speed of a click and consumer behaviors shift in the blink of an eye, dynamic pricing has emerged as the vanguard of modern pricing strategies. It’s a dynamic approach to setting prices for products or services, allowing them to fluctuate in response to real-time market conditions and various external factors.
In this blog post, we will delve into the intricacies of implementing a dynamic pricing model for your business.
Before we start, A Quick Recap of Dynamic Pricing
Dynamic pricing is all about flexibility and adaptability. Instead of adhering to fixed prices, dynamic pricing algorithms continuously assess market variables, customer behavior, and other relevant data to determine the optimal price at any given moment. This approach aims to maximize revenue, profits, or market share, depending on the specific objectives of the business.
You can read more about dynamic pricing in our Ultimate Guide To Dynamic Pricing Strategy In 2023.
Now, let’s delve into the five primary dynamic pricing strategies:
Value-Based Pricing: Leveraging Perceived Worth
Value-based pricing represents a strategic approach that hinges on gauging the perceived worth of a product or service to individual customers and subsequently setting the price at a level congruent with this perceived value.
When to Use: This pricing strategy emerges as an ideal choice for businesses that offer distinct, niche products or services meticulously tailored to cater to a particular and discerning customer base. Additionally, it shines during periods of heightened demand when the opportunity arises to leverage customer willingness to pay a premium in recognition of perceived value.
Example: Luxury brands epitomize the application of value-based pricing. Their offerings are positioned as epitomes of superior quality and status, enabling them to command premium prices commensurate with the perceived prestige they impart.
Cost-Plus Pricing: A Fundamental Approach
Cost-plus pricing is characterized by its straightforwardness, entailing the calculation of the price by appending a fixed percentage or a set amount to the direct costs entailed in the production or provision of a product or service.
When to Use: This pricing strategy finds practicality when an enterprise grapples with constrained capabilities for processing customer data and demands a simplified pricing approach. Cost-plus pricing is the compass that ensures that the costs of production are covered, while simultaneously affording a reasonable profit margin.
Example: Small-scale manufacturing businesses often find solace in cost-plus pricing as it guarantees that their production costs and operational expenditures are addressed, thereby facilitating profit generation.
Time-Based Pricing: Seizing Temporal Opportunities
Time-based pricing takes advantage of temporal opportunities by relying on algorithms to ascertain the price that a customer is likely to be willing to pay at a specific juncture in time. This assessment factors in real-time variables such as prevailing demand, product availability, and even the temporal aspect of the day.
When to Use: This pricing strategy exhibits particular efficacy within industries characterized by volatile market conditions. It empowers businesses to adapt their pricing strategies dynamically, optimizing revenue during periods of heightened demand.
Example: Airlines serve as a quintessential example of time-based pricing. Ticket prices fluctuate based on factors including the timing of booking and the scheduled departure date, ensuring optimal revenue capture.
Competitor-Based Pricing: The Competitive Compass
Competitor-based pricing adheres to a straightforward strategy, wherein algorithms reference the prices established by competitors to either compute an average, set a price lower than top competitors, or align with the pricing set by key rivals.
When to Use: This pricing strategy finds its footing in highly competitive markets, providing businesses with the means to maintain competitiveness and safeguard or expand their market share.
Example: The online retail sector frequently employs competitor-based pricing as a pivotal part of their pricing strategy. Continuous monitoring of the prices of similar products offered by competitors ensures adaptability in pricing.
Inventory-Based Pricing: Striking a Balance
Inventory-based pricing is geared toward optimizing sales and inventory management. It allows businesses to tailor prices in a manner that stimulates the sale of overstocked or slow-moving products, striking a harmonious balance between inventory management and revenue generation.
When to Use: This strategy unfolds its utility for businesses grappling with fluctuating inventory levels, seasonal products, or perishable goods.
Example: Grocery stores adeptly utilize inventory-based pricing, particularly for items nearing their expiration date. This tactical maneuver minimizes waste while maximizing revenue generation.
Now that we’ve established the different types of dynamic pricing strategies you can leverage, let’s take a look at how you can implement dynamic pricing in 6 easy steps.
Dynamic Pricing Implementation: A Step-by-Step Guide
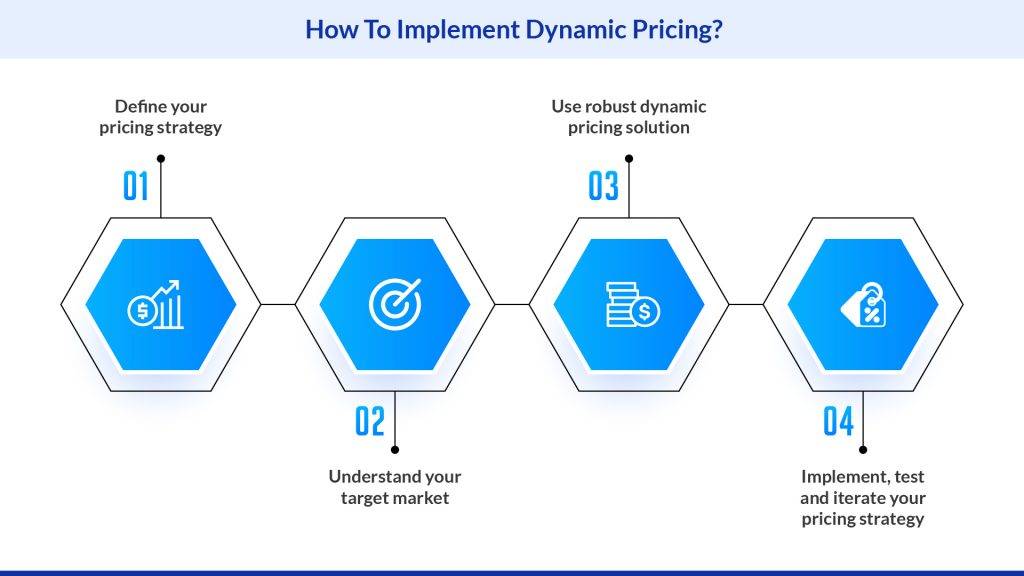
Step 1: Planning the Dynamic Pricing Implementation
The success of dynamic pricing implementation begins with a well-thought-out plan. This initial step sets the stage for the entire process, shaping the strategy that will guide your pricing decisions.
Define Your Pricing Objectives
The first crucial task in planning dynamic pricing implementation is to define your pricing objectives. Without clear objectives, your dynamic pricing strategy can become directionless. Common pricing objectives include:
Maximizing Profitability: This objective focuses on setting prices that maximize profit margins. It’s about finding the balance between revenue and profit, ensuring that each sale contributes significantly to the bottom line.
Increasing Market Share: If your goal is to gain a larger share of the market, your dynamic pricing strategy may prioritize competitive pricing to attract price-sensitive customers and increase your customer base.
Optimizing Revenue: Revenue optimization seeks to strike a balance between maximizing sales volume and maintaining healthy profit margins. It often involves experimenting with different price points to find the sweet spot.
Managing Inventory: Some businesses use dynamic pricing to efficiently manage their inventory. By adjusting prices based on stock levels, you can stimulate sales of excess inventory or slow-moving items.
Enhancing Customer Loyalty: Dynamic pricing can also be used to reward loyal customers with personalized discounts or exclusive offers, fostering customer loyalty.
Understand Your Target Market
To tailor your dynamic pricing strategy effectively, you must have a deep understanding of your target market:
Customer Segmentation: Identify distinct customer segments based on demographics, behavior, and preferences. Dynamic pricing allows you to set prices that resonate with each segment.
Customer Sensitivity: Analyze how price changes affect customer behavior. Some customers are highly price-sensitive and may respond strongly to discounts, while others prioritize quality or convenience.
Market Demand: Study demand patterns in your industry. Are there seasonal fluctuations, special events, or other factors that influence customer demand? Understanding these dynamics can help you fine-tune your pricing strategy.
Data and Technology Assessment
Dynamic pricing relies heavily on data and technology. Assess your organization’s capabilities:
Data Collection: Ensure you have the means to collect relevant data, including customer data, market data, competitor pricing, and historical sales data.
Data Processing: Consider the tools and systems needed to process and analyze the data effectively. Advanced analytics and machine learning models are often employed in dynamic pricing.
Real-time Updates: Dynamic pricing requires real-time updates to respond swiftly to changing market conditions. Evaluate your ability to update prices promptly.
Note: More on this below.
Compliance and Ethical Considerations
Consider the legal and ethical aspects of dynamic pricing:
Regulatory Compliance: Ensure that your dynamic pricing strategies comply with relevant laws and regulations, including pricing transparency and anti-discrimination laws.
Ethical Pricing: Strive for transparency and fairness in your pricing practices.
Implementation Timeline
Set a realistic timeline for implementing dynamic pricing:
Milestones: Define key milestones and checkpoints to monitor progress and ensure that the implementation stays on track.
Testing Phases: Plan for testing phases to assess the effectiveness and accuracy of your dynamic pricing model.
Go-Live Date: Establish a clear go-live date when your dynamic pricing strategy will be fully integrated into your pricing process.
Step 2: Focusing on Data Collection and Exploratory Data Analysis
In this phase, we delve deeper into the critical process of data collection and exploratory data analysis (EDA) as part of your dynamic pricing implementation. This step is the bedrock upon which the success of your dynamic pricing strategy is built, as it provides the empirical foundation for making informed pricing decisions.
Data Collection Strategy: A Holistic Approach
A robust data collection strategy is the cornerstone of dynamic pricing implementation. To ensure comprehensive and effective data collection, consider the following aspects:
Data Sources: Identify and tap into various data sources that are relevant to your industry and business. This includes internal data (e.g., sales records, customer databases) and external data (e.g., market trends, competitor pricing, economic indicators).
Real-Time Data: In the era of dynamic pricing, real-time data is invaluable. Establish mechanisms to collect and integrate real-time data, enabling you to respond swiftly to changing market conditions.
Data Quality: Quality trumps quantity when it comes to data. Implement data quality checks, validation processes, and data cleansing to ensure that the data collected is accurate, complete, and free from errors.
Data Storage: Choose appropriate data storage solutions, whether on-premises or in the cloud, that can handle large volumes of data and facilitate easy access for analysis.
Data Governance and Compliance
Data governance is critical to managing the lifecycle of data, from collection to disposal. Consider the following aspects of data governance:
Data Ownership: Clearly define roles and responsibilities for data ownership within your organization. This includes designating data stewards who are accountable for data quality and compliance.
Data Privacy: Ensure compliance with data privacy regulations such as GDPR or CCPA. Safeguard customer data and adhere to principles of informed consent and data transparency.
Data Retention: Develop policies for data retention and disposal to manage data storage costs and reduce legal risks associated with data retention.
Exploratory Data Analysis (EDA) for Deeper Insights
Exploratory Data Analysis (EDA) is the process of analyzing data sets to summarize their main characteristics, often employing data visualization techniques and statistical measures. EDA serves several key purposes in the context of dynamic pricing:
Data Visualization: Create visual representations of data through charts, graphs, and plots. Visualizations can reveal patterns, outliers, and relationships that might not be immediately apparent in raw data.
Outlier Detection: Identify and investigate outliers in your data. Outliers can provide valuable insights into exceptional events or errors in data collection.
Trend and Seasonality Analysis: EDA allows you to uncover trends and seasonal patterns in historical data. Understanding these patterns is crucial for anticipating future demand fluctuations and optimizing pricing strategies.
Correlation Analysis: Examine relationships between variables. For instance, determine if certain customer demographics correlate with specific purchasing behaviors, helping you tailor pricing strategies for different customer segments.
Data Imputation: Deal with missing data effectively. Impute missing values using appropriate methods, ensuring that your analyses are not compromised by incomplete data.
Advanced Analytics and Predictive Modeling
Consider incorporating advanced analytics and predictive modeling techniques into your EDA process. Machine learning models can provide deeper insights, helping you identify hidden patterns, forecast demand, and predict optimal pricing strategies based on historical data.
Data Security and Ethical Considerations
Data security and ethical considerations are paramount in data collection and EDA:
Data Security: Implement stringent data security measures to protect sensitive customer information, trade secrets, and proprietary data from unauthorized access or breaches.
Ethical Data Usage: Ensure ethical data practices throughout the EDA process. Avoid discriminatory or exploitative use of data and uphold principles of fairness and transparency.
Step 3: Building a Robust Dynamic Pricing Model
Step 3 is a critical juncture in the implementation of dynamic pricing. It is in this phase that you construct the brain of your dynamic pricing strategy: the pricing model. The effectiveness and adaptability of your pricing model will determine how well your business can respond to market changes and customer dynamics. Let’s dive deeper into the intricacies of building a robust dynamic pricing model.
Model Selection
Selecting the appropriate pricing model is akin to choosing the right tool for the job. Machine learning and data-driven models have gained prominence in dynamic pricing. Some popular pricing models include:
Regression Models: Linear and nonlinear regression models are often used to predict the relationship between various factors (e.g., demand, time, competition) and pricing.
Machine Learning Algorithms: Algorithms such as decision trees, random forests, neural networks, and support vector machines can analyze complex relationships and adapt to changing conditions.
Time Series Analysis: For industries with strong seasonality or time-dependent pricing trends, time series analysis can be invaluable in predicting future price fluctuations.
Reinforcement Learning: This AI technique allows the model to learn through interaction with its environment, making it well-suited for pricing decisions that involve continuous adaptation.
The choice of model should align with your specific business needs, the complexity of your pricing environment, and the data available.
Feature Engineering
Feature engineering is the art of selecting, transforming, and creating relevant variables (features) from your dataset to input into the pricing model. Effective feature engineering can significantly enhance the accuracy of your model. Consider factors like:
Historical Sales Data: Past sales performance provides insights into demand trends, seasonality, and market behavior.
Competitor Prices: Monitor and include competitor pricing data to understand how your prices compare in the market.
Customer Segmentation: Segment customers based on demographics, preferences, and purchase history to personalize pricing.
Seasonal Indicators: Incorporate indicators for seasonality, holidays, or special events that impact demand.
Economic Indicators: For some industries, economic factors like inflation, unemployment rates, or consumer sentiment can influence pricing decisions.
Model Training
Training the dynamic pricing model is where the magic happens. You feed historical data into the model, and it uses machine learning techniques to learn patterns, relationships, and behaviors. The model adapts to the data, enabling it to make pricing decisions based on learned insights.
It’s important to ensure that your training data is representative of the market conditions and customer behaviors you want to capture.
Real-Time Data Integration
Dynamic pricing hinges on real-time data. Your model should seamlessly integrate incoming data as it becomes available. This includes updates on market conditions, competitor pricing changes, customer behavior, and any other variables that influence pricing. Real-time integration empowers your model to make timely and responsive pricing decisions.
Pricing Rules and Constraints
While machine learning models can adapt and learn, it’s essential to set clear pricing rules and constraints to guide the model’s decision-making process. These rules may include:
Minimum and Maximum Price Thresholds: Ensuring prices stay within acceptable limits.
Discounting Rules: Defining when and how discounts can be applied.
Customer Segment Strategies: Specifying pricing strategies for different customer segments or personas.
Product-Specific Rules: Tailoring pricing rules to specific products or categories.
Step 4: Testing and Fine-Tuning the Dynamic Pricing Model
As you progress through the implementation of dynamic pricing, Step 4 is the phase where you put your dynamic pricing model to the test. This step is critical for ensuring that your pricing decisions are accurate, responsive, and aligned with your objectives. Let’s explore the intricacies of testing and fine-tuning the dynamic pricing model.
Test Environment Setup
Before conducting tests, establish a controlled testing environment that closely mirrors your actual market conditions. This environment should include:
Historical Data: Use a representative historical dataset that captures a range of market scenarios, customer behaviors, and pricing challenges.
Real-Time Data Simulator: Simulate real-time data feeds to mimic the dynamic nature of your market. This could include pricing changes by competitors, fluctuations in demand, and other relevant variables.
Market Scenarios: Create different market scenarios to assess how your model responds to various conditions, such as sudden spikes in demand, unexpected supply chain disruptions, or aggressive competitive moves.
Scenario Testing
Scenario testing involves subjecting your dynamic pricing model to a series of predefined scenarios to evaluate its performance. These scenarios may include:
Demand Fluctuations: Assess how well the model adjusts prices in response to changes in demand. For example, simulate high-demand periods and observe the model’s pricing decisions.
Competitor Actions: Test the model’s ability to react to changes in competitor pricing. Determine if it can effectively adjust your prices to remain competitive.
Supply Chain Disruptions: Evaluate how the model responds to disruptions in the supply chain, such as delays or shortages. Ensure it can adapt pricing strategies to minimize adverse impacts.
Promotional Events: Test how the model handles promotional events and discounting strategies. Verify that it can execute discounts and promotions effectively.
A/B Testing
A/B testing, also known as split testing, involves comparing the performance of your dynamic pricing model against a control group that follows your existing pricing strategy. This method helps measure the direct impact of dynamic pricing on key performance indicators (KPIs) such as revenue, profit, and market share.
Randomized Groups: Randomly assign customers or market segments to either the dynamic pricing group or the control group. Ensure that the groups are statistically significant.
Measure KPIs: Track KPIs for both groups over a defined period. Compare the results to determine if the dynamic pricing group outperforms the control group.
Iterative Testing: Continuously conduct A/B tests with different variables and pricing strategies to fine-tune your dynamic pricing model.
Feedback Loops
Establish feedback loops to gather input from your pricing team and stakeholders. Encourage regular communication to gather insights, identify issues, and receive feedback on the model’s performance in real-world scenarios. Feedback loops help in continuous improvement and adjustment of your dynamic pricing strategy.
Fine-Tuning and Optimization
Based on the insights gained from testing and feedback, fine-tune your dynamic pricing model. This may involve:
Model Parameter Adjustment: Modify model parameters, weights, or algorithms to enhance accuracy and responsiveness.
Rule Adjustments: Review and update pricing rules and constraints to better align with real-world conditions and pricing objectives.
Data Refinement: Continuously refine and update your data sources and data quality processes to ensure the model is fed accurate and relevant information.
Scenario Simulation: Iterate through scenario testing to verify that the model performs well across various market conditions.
Risk Assessment
Conduct a risk assessment to identify potential vulnerabilities and unintended consequences of your dynamic pricing model. Consider scenarios where the model might lead to extreme pricing fluctuations or customer dissatisfaction. Develop strategies to mitigate these risks, such as implementing pricing caps or incorporating customer feedback mechanisms.
Step 5: Launching the Dynamic Pricing Strategy
The moment has arrived to take your dynamic pricing strategy from the drawing board to the market. Step 5, the launch phase, represents the real-world application of your carefully designed dynamic pricing model. In this critical step, we’ll explore the specific considerations and best practices for a successful launch without repeating the insights from earlier stages.
Pre-launch Preparations
Before rolling out your dynamic pricing strategy, ensure that your preparations are thorough:
Data Infrastructure: Confirm that your data infrastructure is robust, capable of handling real-time data inputs without delays or bottlenecks. Any lag in data processing can disrupt pricing decisions.
Seamless Integration: Integrate your pricing model seamlessly with sales channels, e-commerce platforms, and point-of-sale systems. The model should efficiently update prices and respond to market shifts in real time.
Communication Plan: Develop a clear communication plan to inform your team about the upcoming changes in pricing strategy. Sales and customer service teams should be well-prepared to address customer inquiries.
Soft Launch
Consider a soft launch or phased rollout of your dynamic pricing strategy to:
Fine-Tune: Make any necessary refinements to the pricing model and rules based on early feedback and initial results.
Assess Impact: Evaluate how the strategy influences sales, revenue, and customer behavior within a controlled context.
Build Confidence: Gain confidence in the strategy’s effectiveness before full-scale implementation, reducing the risk of unforeseen challenges.
Customer Communication
Transparent and proactive communication with customers is paramount, especially when prices are subject to frequent changes. Strategies for effective communication include:
Price Notifications: Informing customers about significant price adjustments to manage expectations.
Value Proposition: Reinforce the value customers receive despite price fluctuations, highlighting product quality or exceptional service.
Personalization: Customize offers and discounts for loyal customers to mitigate potential dissatisfaction.
Step 6: Measuring the Effectiveness of Dynamic Pricing Strategy
After meticulous planning, data collection, model development, testing, and the official launch of your dynamic pricing strategy, it’s time to turn your attention to Step 6: Measuring the Effectiveness of your dynamic pricing strategy. This phase is crucial for assessing the impact of your strategy, making necessary adjustments, and ensuring that your pricing objectives are being met.
Key Performance Indicators (KPIs)
To gauge the effectiveness of your dynamic pricing strategy, you’ll need to establish and monitor Key Performance Indicators (KPIs) that align with your pricing objectives. Here are some common KPIs:
- Revenue Growth: Monitor changes in overall revenue and assess whether your dynamic pricing strategy is contributing to revenue growth.
- Profit Margin: Evaluate the impact of dynamic pricing on profit margins. Ensure that increased sales volume is not coming at the expense of profitability.
- Market Share: Measure your market share to determine whether competitive pricing strategies have been successful in gaining a larger share of the market.
- Customer Retention: Track customer retention rates to assess whether dynamic pricing has enhanced customer loyalty and encouraged repeat business.
- Price Elasticity: Calculate price elasticity to understand how changes in pricing affect customer demand. This information can help you fine-tune your pricing strategies.
- Competitive Position: Continuously monitor your competitive position by assessing how your prices compare with competitors’ prices.
Customer Feedback and Surveys
Direct feedback from your customers can offer valuable insights into the effectiveness of your dynamic pricing strategy. Conduct surveys or collect feedback through customer service channels to understand how customers perceive your pricing changes. Their opinions can reveal whether the strategy aligns with their expectations and influences their purchase decisions.
Continuous Monitoring and Adaptation
Dynamic pricing is an ongoing process. Market conditions, customer behaviors, and competitive landscapes can change rapidly. Therefore, it’s essential to continuously monitor your dynamic pricing strategy and make necessary adaptations in real time.
Utilize data analytics tools and dashboards to track KPIs, monitor competitor pricing, and stay informed about market trends. Automated alerts can notify you when certain pricing thresholds or conditions are met, enabling swift responses.
Adjustments and Optimization
Based on the insights gained from the measurement phase, be prepared to make adjustments and optimizations to your dynamic pricing strategy. This may involve tweaking pricing rules, altering pricing models, or refining your segmentation strategy. Keep in mind that optimization is an iterative process, and ongoing fine-tuning is often necessary to maximize results.
Implement dynamic pricing strategy with Flipkart Commerce Cloud
Flipkart Commerce Cloud’s Dynamic Pricing engine is not just a pricing tool; it’s a comprehensive solution designed to empower retailers and brands in the ever-evolving landscape of commerce. With guidance from seasoned retail experts, a wealth of experience spanning over 14 years, and a relentless emphasis on the foundation of quality data, FCC’s Dynamic Pricing engine provides the competitive edge needed in today’s market.
This pricing optimizer, driven by proprietary algorithms that combine game theory and traditional statistics, ensures that your pricing strategy is not just adaptive but also optimized for maximum profitability. With a robust rule engine and the ability to manage multiple categories and SKUs effortlessly, FCC equips your business to thrive, expand, and maximize margins. With FCC, dynamic pricing becomes a strategic advantage, enabling you to stay agile, competitive, and successful in the world of retail.
If you find this process daunting, don’t worry; our experts are here to assist you. Get in touch with us today!